Generative AI and other tools, for the future of business
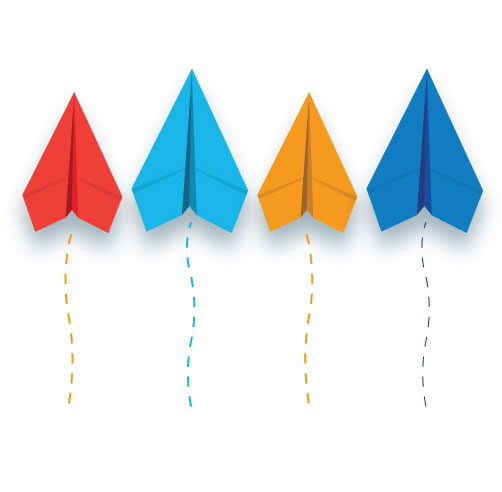
What is Artificial Intelligence (AI)
The imitation of human decision-making processes by computer systems is known as artificial intelligence. This concept has always fascinated people in movies like “Short Circuit,” “Terminator” (my personal favorite), “I, Robot,” or “Wall-E.”. Although a great deal of creativity has gone into creating human like robots with emotions and strong decision-making abilities, the majority of artificial intelligence in use today is software that mimics human intellect. Applications that are hosted on the cloud, enterprise apps, and even mobile apps demonstrate this.
Overview of Machine Learning (ML)
You might wonder, “How do machines learn?” Well, in machine learning, computers are trained to identify patterns in historical data. When new data comes in, this pattern recognition helps them make predictions, which can be used to inform business decisions.
To train models in machine learning, we use datasets containing features (input) and labels (output). The goal is for the algorithm to find a formula that predicts labels based on features. Then, when new data comes in, the algorithm applies this formula to make predictions.
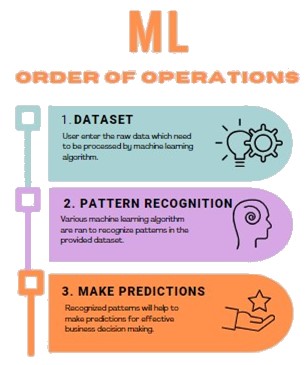
Machine Learning Order of Operations
A model must be trained using high-quality data sets. It is a lot like finding the appropriate line to fit a set of discrete data points, very similar to curve fitting.Various equations can provide a more precise fit for the curve.
- The red line in the above image represents the mean line
- The green line a slightly better fit
- The purple line the best fit.

Curve Fitting
Empirical risk minimization is the process of adding new data to the model so that it fits better and is optimized.
This is merely a basic illustration; you can imagine the set of issues being multiplied numerous times and discover applications in a variety of industries, such as data extraction and analysis, fraud detection, sales pattern identification, and product recommendation to clients.
Here are a few commonly used tools that leverage machine learning.
- Amazon Sagemaker makes it simple and quick to begin using machine learning. The solutions allow for one-click deployment and fine-tuning of over 150 widely-used open-source models, including image classification, object identification, and natural language processing. They are totally customizable.
- Microsoft Azure Machine Learning is a cloud platform with tools for building, training, and deploying machine learning models. It’s scalable and lets data scientists and developers collaborate to experiment with algorithms, handle data, and deploy models.
- Google Cloud AI Platform is a service for building and deploying machine learning models in the cloud, offering flexibility and collaboration for data scientists and developers.
- IBM Watson AI provides a range of AI tools and services to help businesses use artificial intelligence effectively, including features like natural language processing and predictive analytics for problem-solving and innovation.
What is Generative AI?
When dealing with larger and more varied datasets, like patient or customer data, which may contain a variety of information types like photos or data from several sources, the straightforward curve fitting approach that was previously mentioned might become more difficult.
While generative AI uses generative models and unsupervised learning, traditional AI depends on supervised learning and models trained with particular data. This distinction is important because Retrieval Augmented Generation (RAG) and other methods are needed to filter out unnecessary outputs that Generative AI may produce.
Depending on the intended result and the application domain, the complexity of AI models can vary significantly, leading to a variety of models that are suited to particular domains and applications.
Here are a few commonly used tools that leverage Generative AI.
- Amazon CodeWhisperer is an example of a generative AI application. It can generate code suggestions in real time based on your comments and existing code.
- ChatGPT an AI language model developed by OpenAI. It can answer questions and generate human-like responses from text prompts.
- Google Bard an AI Chatbot. It can answer questions and generate human-like responses from text prompts.
Relation between Artificial Intelligence, Machine Learning, Deep Learning and Generative AI
Artificial intelligence (AI) comprises machine learning, which includes deep learning, and generative AI, which is focused on creating new data samples, which falls under machine learning.
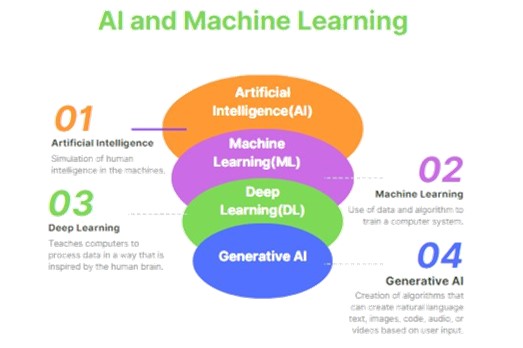
AI and Machine Learning
Generative AI use cases
Generative AI holds immense potential to revolutionize various sectors of the world economy. Here’s how it can be applied in key areas:
Manufacturing
Here are some ways that generative AI benefits manufacturing sector:
- Instant Equipment Diagnostics: By using past data, predictive AI may identify equipment malfunctions in real time and prescribe maintenance procedures like input corrections, repairs, or suggested replacement parts.
- Optimization of product design: Manufacturers can find the most optimal, effective, and economical solutions by using generative AI, which can swiftly develop and evaluate many design choices.
- AI-driven maintenance agents: Product manuals, troubleshooting instructions, and maintenance notes can be used to educate generative conversational agents so they can provide workers with quick technical support and save downtime.
Finance
Here are some of the ways that generative AI benefits finance:
- Fraud detection: AI is able to mimic different patterns of money laundering, which aids in the detection of fraud.
- Portfolio management: AI’s ability to model different market conditions will aid in building and maintaining a robust portfolio for investors.
- Debt collection: AI is capable of producing efficient negotiating and communication plans.
Entertainment and media
Here are some ways that generative AI benefits media and entertainment:
- Content generation:AI can write dialogue, plots, and scripts for movies, TV series, and video games.
- Virtual reality:Interactive virtual worlds for simulations or games can be produced with generative AI.
- Art and design:Generative AI is capable of producing imaginative and inventive artwork.
Medical Care
Generative AI helps the medical field in the following ways:
- Clinical notes: By examining patient-doctor interactions, AI is able to automatically produce clinical notes.
- Prescription medicine: AI is able to recommend medications and treatment regimens depending on a patient’s lifestyle, genetics, and the course of their illness.
- Medical pictures: AI is capable of producing images from MRIs, CT scans, and X-rays, which can aid in more accurate diagnosis.

Generative AI Use Cases
Advantages of generative AI
- Accurate simulation: Art, healthcare, banking, and the automotive industries are just a few of the industries that can benefit from the use of generative AI to produce realistic simulations.
- Original and captivating content: Generative AI produces captivating images, films, narratives, scripts, and conversation for motion pictures, television series, and video games. Many cutting-edge methods for reducing manual creative effort are offered by generative AI.
- Customization: Generative AI tailors the customer experience by analyzing individual customer data and offering recommendations and personalized offers based on their preferences, thereby enhancing the customer experience through customization.
- Increased productivity and efficiency: Generative AI automates processes that once needed human interaction, enabling businesses to make better use of their resources and increase productivity and efficiency. One example of this efficiency gain is the generative AI program Amazon CodeWhisperer, which offers real-time code ideas based on comments and current code.
- Elevated customer satisfaction: Generative AI tailors recommendations and offers based on individual preferences, thereby enhancing customer satisfaction through personalized experiences.
Disadvantages of Generative AI
- Compliance and regulatory risks: Users may unknowingly share Personally Identifiable Information (PII) with Generative AI platforms, leading to serious compliance and regulatory violations.
- Social and privacy concerns: Unwanted content produced by AI may have a negative effect on particular groups of people or communities.
- Potential for misuse: Malevolent individuals might utilize AI-generated data to propagate false information, aiding in the spread of fake news and unsettling the public.
- Data vulnerability: People may unintentionally give sensitive information—such user IDs or API keys—to Generative AI apps like ChatGPT. If this information is sent over unsecure networks, it can be captured by attackers and harm the reputation of the company.
Disadvantages of Generative AI
At Stovl, we focus on specific verticals and types of application sets, providing custom-fit solutions tailored to address our customers’ needs effectively. Some of our solutions include:
- Specy: A Document Reading Assistant designed to enhance productivity and facilitate seamless information retrieval.
- AsqueMe: An efficient Customer Experience (CX) Platform that transforms FAQ handling across various industries.
- WeBOT: A VoiceBot redefining Customer Support and Operations within the emerging tech landscape of rural India.
- Ctrials: Conversational AI-enabled solution for facilitating Clinical Trial matching processes.

What We Offer @Stovl
Conclusion
The global economy could undergo radical transformations thanks to generative AI. Clinical notes, medications, and medical photographs can all be created automatically. Screenplays, dialogue, and tales for movies, TV series, and video games may all be produced with it. It offers excellent capabilities for generating code, content creation, and content summarizing. Companies should use generative AI to differentiate themselves in a world that is evolving quickly.
Comments are closed.